PowerCo - EDA#
The Datasets#
ml_case_training_output.csv
named as pco_output
contains:
id: contact id
churned: has the client churned over the next 3 months
ml_case_training_hist_data.csv
named as pco_hist
contains the history of energy and power consumption per client:
id: contact id
price_date: reference date
price_p1_var: price of energy for the 1st period
price_p2_var: price of energy for the 2nd
periodprice_p3_var: price of energy for the 3rd period
price_p1_fix: price of power for the 1st period
price_p2_fix: price of power for the 2nd period
price_p3_fix: price of power for the 3rd period
ml_case_training_data.csv
contains:
id: contact id
activity_new: category of the company’s activity.
campaign_disc_elec: code of the electricity campaign the customer last subscribed to.
channel_sales: code of the sales channel
cons_12m: electricity consumption of the past 12 months
cons_gas_12m: gas consumption of the past 12 months
cons_last_month: electricity consupmtion of the last month
date_activ: date of activation of the contract
date_end: registered date of the end of the contract
date_first_activ: date of first contract of the client
date_modif_prod: date of last modification of the product
date_renewal: date of the next contract renewal
forecast_base_bill_ele: forecasted electricity bill baseline for next month
forecast_base_bill_year: forecasted electricity bill baseline for calendar year
forecast_bill_12m: forecasted electricity bill baseline for 12 months
forecast_cons: forecasted electricity consumption for next month
forecast_cons_12m: forecasted electricity consumption for next 12 months
forecast_cons_year: forecasted electricity consumption for next calendar year
forecast_discount_energy: forecasted value of current discount
forecast_meter_rent_12m: forecasted bill of meter rental for the next 12 months
forecast_price_energy_p1: forecasted energy price for 1st period
forecast_price_energy_p2: forecasted energy price for 2nd period
forecast_price_pow_p1: forecasted power price for 1st period
has_gas: indicated if client is also a gas client
imp_cons: current paid consumption
margin_gross_pow_ele: gross margin on power subscription
margin_net_pow_ele: net margin on power subscription
nb_prod_act: number of active products and services
net_margin: total net margin
num_years_antig: antiquity of the client (in number of years)
origin_up: code of the electricity campaign the customer first subscribed to
pow_max: subscribed power
Import Libraries#
import pandas as pd
import numpy as np
import seaborn as sns
import matplotlib.pyplot as plt
# Show plots in jupyter notebook
%matplotlib inline
import missingno as msno
from scipy.stats import zscore as zscore
import warnings
warnings.filterwarnings("ignore")
# Set maximum number of columns to be displayed
pd.set_option('display.max_columns', 100)
Load Data#
# list of dates
dt_lst = ['date_activ','date_end','date_first_activ','date_modif_prod','date_renewal']
pco_main = pd.read_csv('data/ml_case_training_data.csv', parse_dates=dt_lst)
pco_hist = pd.read_csv('data/ml_case_training_hist_data.csv', parse_dates=['price_date'])
pco_output = pd.read_csv('data/ml_case_training_output.csv')
pd.set_option('display.max_columns',None)
Main Dataset#
pco_main.head()
id | activity_new | campaign_disc_ele | channel_sales | cons_12m | cons_gas_12m | cons_last_month | date_activ | date_end | date_first_activ | date_modif_prod | date_renewal | forecast_base_bill_ele | forecast_base_bill_year | forecast_bill_12m | forecast_cons | forecast_cons_12m | forecast_cons_year | forecast_discount_energy | forecast_meter_rent_12m | forecast_price_energy_p1 | forecast_price_energy_p2 | forecast_price_pow_p1 | has_gas | imp_cons | margin_gross_pow_ele | margin_net_pow_ele | nb_prod_act | net_margin | num_years_antig | origin_up | pow_max | |
---|---|---|---|---|---|---|---|---|---|---|---|---|---|---|---|---|---|---|---|---|---|---|---|---|---|---|---|---|---|---|---|---|
0 | 48ada52261e7cf58715202705a0451c9 | esoiiifxdlbkcsluxmfuacbdckommixw | NaN | lmkebamcaaclubfxadlmueccxoimlema | 309275 | 0 | 10025 | 2012-11-07 | 2016-11-06 | NaT | 2012-11-07 | 2015-11-09 | NaN | NaN | NaN | NaN | 26520.30 | 10025 | 0.0 | 359.29 | 0.095919 | 0.088347 | 58.995952 | f | 831.8 | -41.76 | -41.76 | 1 | 1732.36 | 3 | ldkssxwpmemidmecebumciepifcamkci | 180.000 |
1 | 24011ae4ebbe3035111d65fa7c15bc57 | NaN | NaN | foosdfpfkusacimwkcsosbicdxkicaua | 0 | 54946 | 0 | 2013-06-15 | 2016-06-15 | NaT | NaT | 2015-06-23 | NaN | NaN | NaN | NaN | 0.00 | 0 | 0.0 | 1.78 | 0.114481 | 0.098142 | 40.606701 | t | 0.0 | 25.44 | 25.44 | 2 | 678.99 | 3 | lxidpiddsbxsbosboudacockeimpuepw | 43.648 |
2 | d29c2c54acc38ff3c0614d0a653813dd | NaN | NaN | NaN | 4660 | 0 | 0 | 2009-08-21 | 2016-08-30 | NaT | 2009-08-21 | 2015-08-31 | NaN | NaN | NaN | NaN | 189.95 | 0 | 0.0 | 16.27 | 0.145711 | 0.000000 | 44.311378 | f | 0.0 | 16.38 | 16.38 | 1 | 18.89 | 6 | kamkkxfxxuwbdslkwifmmcsiusiuosws | 13.800 |
3 | 764c75f661154dac3a6c254cd082ea7d | NaN | NaN | foosdfpfkusacimwkcsosbicdxkicaua | 544 | 0 | 0 | 2010-04-16 | 2016-04-16 | NaT | 2010-04-16 | 2015-04-17 | NaN | NaN | NaN | NaN | 47.96 | 0 | 0.0 | 38.72 | 0.165794 | 0.087899 | 44.311378 | f | 0.0 | 28.60 | 28.60 | 1 | 6.60 | 6 | kamkkxfxxuwbdslkwifmmcsiusiuosws | 13.856 |
4 | bba03439a292a1e166f80264c16191cb | NaN | NaN | lmkebamcaaclubfxadlmueccxoimlema | 1584 | 0 | 0 | 2010-03-30 | 2016-03-30 | NaT | 2010-03-30 | 2015-03-31 | NaN | NaN | NaN | NaN | 240.04 | 0 | 0.0 | 19.83 | 0.146694 | 0.000000 | 44.311378 | f | 0.0 | 30.22 | 30.22 | 1 | 25.46 | 6 | kamkkxfxxuwbdslkwifmmcsiusiuosws | 13.200 |
pco_main.info()
<class 'pandas.core.frame.DataFrame'>
RangeIndex: 16096 entries, 0 to 16095
Data columns (total 32 columns):
# Column Non-Null Count Dtype
--- ------ -------------- -----
0 id 16096 non-null object
1 activity_new 6551 non-null object
2 campaign_disc_ele 0 non-null float64
3 channel_sales 11878 non-null object
4 cons_12m 16096 non-null int64
5 cons_gas_12m 16096 non-null int64
6 cons_last_month 16096 non-null int64
7 date_activ 16096 non-null datetime64[ns]
8 date_end 16094 non-null datetime64[ns]
9 date_first_activ 3508 non-null datetime64[ns]
10 date_modif_prod 15939 non-null datetime64[ns]
11 date_renewal 16056 non-null datetime64[ns]
12 forecast_base_bill_ele 3508 non-null float64
13 forecast_base_bill_year 3508 non-null float64
14 forecast_bill_12m 3508 non-null float64
15 forecast_cons 3508 non-null float64
16 forecast_cons_12m 16096 non-null float64
17 forecast_cons_year 16096 non-null int64
18 forecast_discount_energy 15970 non-null float64
19 forecast_meter_rent_12m 16096 non-null float64
20 forecast_price_energy_p1 15970 non-null float64
21 forecast_price_energy_p2 15970 non-null float64
22 forecast_price_pow_p1 15970 non-null float64
23 has_gas 16096 non-null object
24 imp_cons 16096 non-null float64
25 margin_gross_pow_ele 16083 non-null float64
26 margin_net_pow_ele 16083 non-null float64
27 nb_prod_act 16096 non-null int64
28 net_margin 16081 non-null float64
29 num_years_antig 16096 non-null int64
30 origin_up 16009 non-null object
31 pow_max 16093 non-null float64
dtypes: datetime64[ns](5), float64(16), int64(6), object(5)
memory usage: 3.9+ MB
# Percentage of nullity by column
missing_perc = pco_main.isnull().mean() * 100
print('Percentage of Missing Values:\n', missing_perc)
Percentage of Missing Values:
id 0.000000
activity_new 59.300447
campaign_disc_ele 100.000000
channel_sales 26.205268
cons_12m 0.000000
cons_gas_12m 0.000000
cons_last_month 0.000000
date_activ 0.000000
date_end 0.012425
date_first_activ 78.205765
date_modif_prod 0.975398
date_renewal 0.248509
forecast_base_bill_ele 78.205765
forecast_base_bill_year 78.205765
forecast_bill_12m 78.205765
forecast_cons 78.205765
forecast_cons_12m 0.000000
forecast_cons_year 0.000000
forecast_discount_energy 0.782803
forecast_meter_rent_12m 0.000000
forecast_price_energy_p1 0.782803
forecast_price_energy_p2 0.782803
forecast_price_pow_p1 0.782803
has_gas 0.000000
imp_cons 0.000000
margin_gross_pow_ele 0.080765
margin_net_pow_ele 0.080765
nb_prod_act 0.000000
net_margin 0.093191
num_years_antig 0.000000
origin_up 0.540507
pow_max 0.018638
dtype: float64
# Descriptive statistics
pco_main.describe()
campaign_disc_ele | cons_12m | cons_gas_12m | cons_last_month | date_activ | date_end | date_first_activ | date_modif_prod | date_renewal | forecast_base_bill_ele | forecast_base_bill_year | forecast_bill_12m | forecast_cons | forecast_cons_12m | forecast_cons_year | forecast_discount_energy | forecast_meter_rent_12m | forecast_price_energy_p1 | forecast_price_energy_p2 | forecast_price_pow_p1 | imp_cons | margin_gross_pow_ele | margin_net_pow_ele | nb_prod_act | net_margin | num_years_antig | pow_max | |
---|---|---|---|---|---|---|---|---|---|---|---|---|---|---|---|---|---|---|---|---|---|---|---|---|---|---|---|
count | 0.0 | 1.609600e+04 | 1.609600e+04 | 1.609600e+04 | 16096 | 16094 | 3508 | 15939 | 16056 | 3508.000000 | 3508.000000 | 3508.000000 | 3508.000000 | 16096.000000 | 16096.000000 | 15970.000000 | 16096.000000 | 15970.000000 | 15970.000000 | 15970.000000 | 16096.000000 | 16083.000000 | 16083.000000 | 16096.000000 | 16081.000000 | 16096.000000 | 16093.000000 |
mean | NaN | 1.948044e+05 | 3.191164e+04 | 1.946154e+04 | 2011-01-17 08:58:18.011928320 | 2016-07-27 11:36:49.568783360 | 2011-06-19 20:20:23.261117440 | 2012-12-14 18:33:40.553359872 | 2015-07-20 21:56:30.134529280 | 335.843857 | 335.843857 | 3837.441866 | 206.845165 | 2370.555949 | 1907.347229 | 0.991547 | 70.309945 | 0.135901 | 0.052951 | 43.533496 | 196.123447 | 22.462276 | 21.460318 | 1.347788 | 217.987028 | 5.030629 | 20.604131 |
min | NaN | -1.252760e+05 | -3.037000e+03 | -9.138600e+04 | 2000-07-25 00:00:00 | 2006-08-26 00:00:00 | 2001-01-10 00:00:00 | 2000-07-25 00:00:00 | 2013-06-26 00:00:00 | -364.940000 | -364.940000 | -2503.480000 | 0.000000 | -16689.260000 | -85627.000000 | 0.000000 | -242.960000 | 0.000000 | 0.000000 | -0.122184 | -9038.210000 | -525.540000 | -615.660000 | 1.000000 | -4148.990000 | 1.000000 | 1.000000 |
25% | NaN | 5.906250e+03 | 0.000000e+00 | 0.000000e+00 | 2010-01-12 00:00:00 | 2016-04-28 00:00:00 | 2010-08-04 18:00:00 | 2010-08-10 00:00:00 | 2015-04-19 00:00:00 | 0.000000 | 0.000000 | 1158.175000 | 0.000000 | 513.230000 | 0.000000 | 0.000000 | 16.230000 | 0.115237 | 0.000000 | 40.606701 | 0.000000 | 11.960000 | 11.950000 | 1.000000 | 51.970000 | 4.000000 | 12.500000 |
50% | NaN | 1.533250e+04 | 0.000000e+00 | 9.010000e+02 | 2011-03-04 00:00:00 | 2016-07-30 00:00:00 | 2011-10-28 00:00:00 | 2013-05-01 00:00:00 | 2015-07-24 00:00:00 | 162.955000 | 162.955000 | 2187.230000 | 42.215000 | 1179.160000 | 378.000000 | 0.000000 | 19.440000 | 0.142881 | 0.086163 | 44.311378 | 44.465000 | 21.090000 | 20.970000 | 1.000000 | 119.680000 | 5.000000 | 13.856000 |
75% | NaN | 5.022150e+04 | 0.000000e+00 | 4.127000e+03 | 2012-04-26 00:00:00 | 2016-10-31 00:00:00 | 2012-06-22 06:00:00 | 2015-05-24 00:00:00 | 2015-10-30 00:00:00 | 396.185000 | 396.185000 | 4246.555000 | 228.117500 | 2692.077500 | 1994.250000 | 0.000000 | 131.470000 | 0.146348 | 0.098837 | 44.311378 | 218.090000 | 29.640000 | 29.640000 | 1.000000 | 275.810000 | 6.000000 | 19.800000 |
max | NaN | 1.609711e+07 | 4.188440e+06 | 4.538720e+06 | 2014-09-01 00:00:00 | 2017-06-13 00:00:00 | 2014-09-01 00:00:00 | 2016-01-29 00:00:00 | 2016-01-28 00:00:00 | 12566.080000 | 12566.080000 | 81122.630000 | 9682.890000 | 103801.930000 | 175375.000000 | 50.000000 | 2411.690000 | 0.273963 | 0.195975 | 59.444710 | 15042.790000 | 374.640000 | 374.640000 | 32.000000 | 24570.650000 | 16.000000 | 500.000000 |
std | NaN | 6.795151e+05 | 1.775885e+05 | 8.235676e+04 | NaN | NaN | NaN | NaN | NaN | 649.406000 | 649.406000 | 5425.744327 | 455.634288 | 4035.085664 | 5257.364759 | 5.160969 | 79.023251 | 0.026252 | 0.048617 | 5.212252 | 494.366979 | 23.700883 | 27.917349 | 1.459808 | 366.742030 | 1.676101 | 21.772421 |
Observations#
14 columns have negative minimum values.
campaign_disc_ele
column is missing completely.activity_new
column is missing 59.3%.The
date_first_active
,forecast_base_bill_ele
,forecast_base_bill_year
,forecast_bill_12m
, andforecast_cons
columns are each missing 78.2%.
The History Dataset#
pco_hist.head()
id | price_date | price_p1_var | price_p2_var | price_p3_var | price_p1_fix | price_p2_fix | price_p3_fix | |
---|---|---|---|---|---|---|---|---|
0 | 038af19179925da21a25619c5a24b745 | 2015-01-01 | 0.151367 | 0.0 | 0.0 | 44.266931 | 0.0 | 0.0 |
1 | 038af19179925da21a25619c5a24b745 | 2015-02-01 | 0.151367 | 0.0 | 0.0 | 44.266931 | 0.0 | 0.0 |
2 | 038af19179925da21a25619c5a24b745 | 2015-03-01 | 0.151367 | 0.0 | 0.0 | 44.266931 | 0.0 | 0.0 |
3 | 038af19179925da21a25619c5a24b745 | 2015-04-01 | 0.149626 | 0.0 | 0.0 | 44.266931 | 0.0 | 0.0 |
4 | 038af19179925da21a25619c5a24b745 | 2015-05-01 | 0.149626 | 0.0 | 0.0 | 44.266931 | 0.0 | 0.0 |
pco_hist.info()
<class 'pandas.core.frame.DataFrame'>
RangeIndex: 193002 entries, 0 to 193001
Data columns (total 8 columns):
# Column Non-Null Count Dtype
--- ------ -------------- -----
0 id 193002 non-null object
1 price_date 193002 non-null datetime64[ns]
2 price_p1_var 191643 non-null float64
3 price_p2_var 191643 non-null float64
4 price_p3_var 191643 non-null float64
5 price_p1_fix 191643 non-null float64
6 price_p2_fix 191643 non-null float64
7 price_p3_fix 191643 non-null float64
dtypes: datetime64[ns](1), float64(6), object(1)
memory usage: 11.8+ MB
# Percentage of nullity by column
missing_perc = pco_hist.isnull().mean() * 100
print('Percentage of Missing Values:\n', missing_perc)
Percentage of Missing Values:
id 0.000000
price_date 0.000000
price_p1_var 0.704138
price_p2_var 0.704138
price_p3_var 0.704138
price_p1_fix 0.704138
price_p2_fix 0.704138
price_p3_fix 0.704138
dtype: float64
# Descriptive statistics
pco_hist.describe()
price_date | price_p1_var | price_p2_var | price_p3_var | price_p1_fix | price_p2_fix | price_p3_fix | |
---|---|---|---|---|---|---|---|
count | 193002 | 191643.000000 | 191643.000000 | 191643.000000 | 191643.000000 | 191643.000000 | 191643.000000 |
mean | 2015-06-16 12:50:49.933161216 | 0.140991 | 0.054412 | 0.030712 | 43.325546 | 10.698201 | 6.455436 |
min | 2015-01-01 00:00:00 | 0.000000 | 0.000000 | 0.000000 | -0.177779 | -0.097752 | -0.065172 |
25% | 2015-04-01 00:00:00 | 0.125976 | 0.000000 | 0.000000 | 40.728885 | 0.000000 | 0.000000 |
50% | 2015-07-01 00:00:00 | 0.146033 | 0.085483 | 0.000000 | 44.266930 | 0.000000 | 0.000000 |
75% | 2015-10-01 00:00:00 | 0.151635 | 0.101780 | 0.072558 | 44.444710 | 24.339581 | 16.226389 |
max | 2015-12-01 00:00:00 | 0.280700 | 0.229788 | 0.114102 | 59.444710 | 36.490692 | 17.458221 |
std | NaN | 0.025117 | 0.050033 | 0.036335 | 5.437952 | 12.856046 | 7.782279 |
Observations#
price_p1_var
,price_p2_var
,price_p3_var
,price_p1_fix
,price_p2_fix
,price_p3_fix
are missing 70.4% values.price_p1_fix
,price_p2_fix
,price_p3_fix
contain negative values, which doesn’t make sense for price of power.
The Output Dataset#
pco_output.head()
id | churn | |
---|---|---|
0 | 48ada52261e7cf58715202705a0451c9 | 0 |
1 | 24011ae4ebbe3035111d65fa7c15bc57 | 1 |
2 | d29c2c54acc38ff3c0614d0a653813dd | 0 |
3 | 764c75f661154dac3a6c254cd082ea7d | 0 |
4 | bba03439a292a1e166f80264c16191cb | 0 |
pco_output.info()
<class 'pandas.core.frame.DataFrame'>
RangeIndex: 16096 entries, 0 to 16095
Data columns (total 2 columns):
# Column Non-Null Count Dtype
--- ------ -------------- -----
0 id 16096 non-null object
1 churn 16096 non-null int64
dtypes: int64(1), object(1)
memory usage: 251.6+ KB
# Percentage of nullity by column
missing_perc = pco_output.isnull().mean() * 100
print('Percentage of Missing Values:\n', missing_perc)
Percentage of Missing Values:
id 0.0
churn 0.0
dtype: float64
# Descriptive statistics
pco_output.describe()
churn | |
---|---|
count | 16096.000000 |
mean | 0.099093 |
std | 0.298796 |
min | 0.000000 |
25% | 0.000000 |
50% | 0.000000 |
75% | 0.000000 |
max | 1.000000 |
Observations#
Complete dataset.
Data Cleaning and Imputation#
Missing Data#
Types of missingness#
Missing Completely at Random (MCAR)
Missingness has no relationship between any values, observed or missing
Missing at Random (MAR)
There is a systematic relationship between missingness and other observed data, but not the missing data
Missing Not at Random (MNAR)
There is a relationship between missingness and its values, missing or non-missing
The History Dataset#
# Identify negative columns
negative_cols = ['price_p1_fix','price_p2_fix','price_p3_fix']
# Convert to positive the negative columns in pco_hist
pco_hist[negative_cols] = pco_hist[negative_cols].apply(abs)
pco_hist.describe()
price_date | price_p1_var | price_p2_var | price_p3_var | price_p1_fix | price_p2_fix | price_p3_fix | |
---|---|---|---|---|---|---|---|
count | 193002 | 191643.000000 | 191643.000000 | 191643.000000 | 191643.000000 | 191643.000000 | 191643.000000 |
mean | 2015-06-16 12:50:49.933161216 | 0.140991 | 0.054412 | 0.030712 | 43.325563 | 10.698210 | 6.455443 |
min | 2015-01-01 00:00:00 | 0.000000 | 0.000000 | 0.000000 | 0.000000 | 0.000000 | 0.000000 |
25% | 2015-04-01 00:00:00 | 0.125976 | 0.000000 | 0.000000 | 40.728885 | 0.000000 | 0.000000 |
50% | 2015-07-01 00:00:00 | 0.146033 | 0.085483 | 0.000000 | 44.266930 | 0.000000 | 0.000000 |
75% | 2015-10-01 00:00:00 | 0.151635 | 0.101780 | 0.072558 | 44.444710 | 24.339581 | 16.226389 |
max | 2015-12-01 00:00:00 | 0.280700 | 0.229788 | 0.114102 | 59.444710 | 36.490692 | 17.458221 |
std | NaN | 0.025117 | 0.050033 | 0.036335 | 5.437816 | 12.856039 | 7.782273 |
# Visualize the completeness of the dataframe
msno.bar(pco_hist)
plt.show()
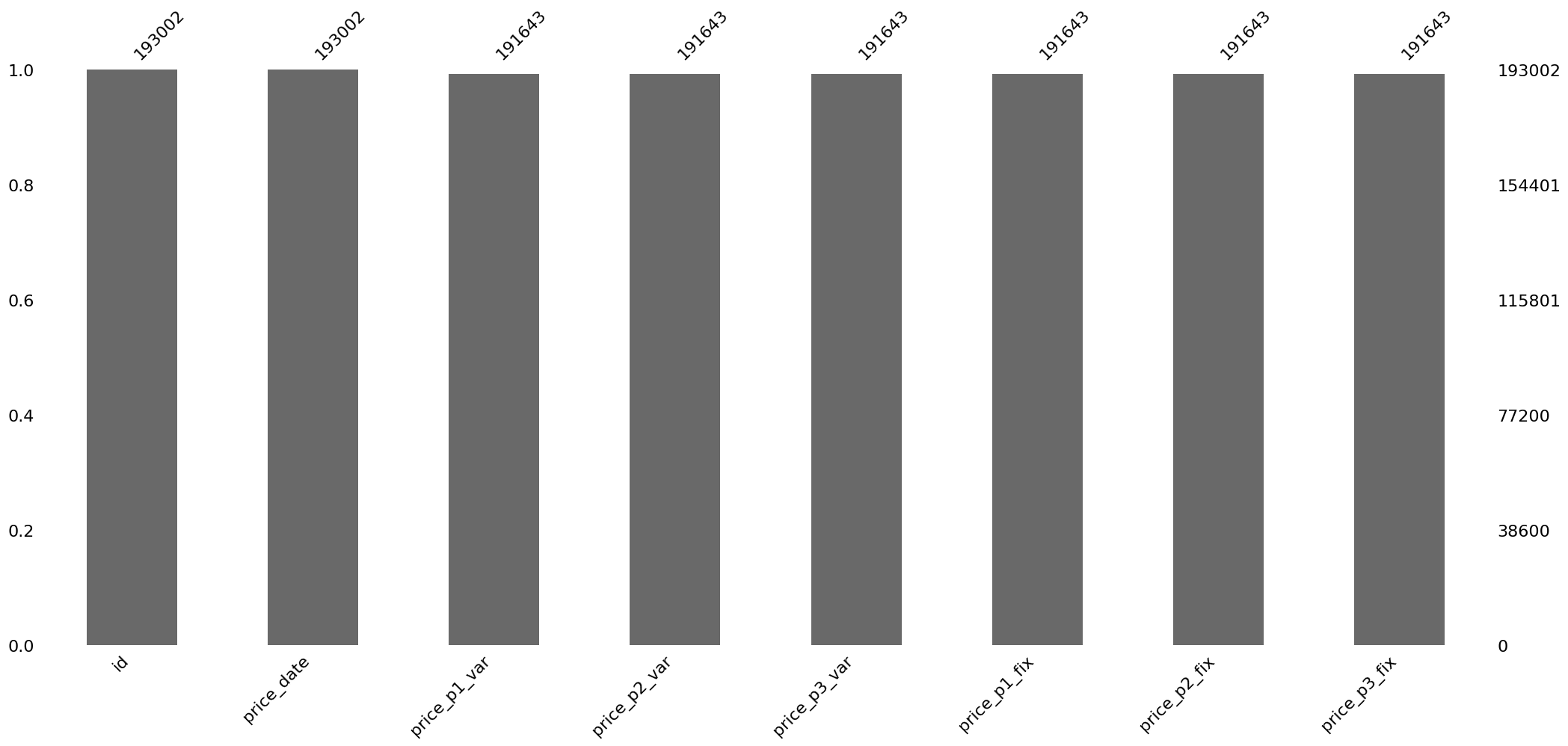
# Visualize the locations of the missing values of the dataset
sorted = pco_hist.sort_values(by = ['id','price_date'])
msno.matrix(sorted)
plt.show()
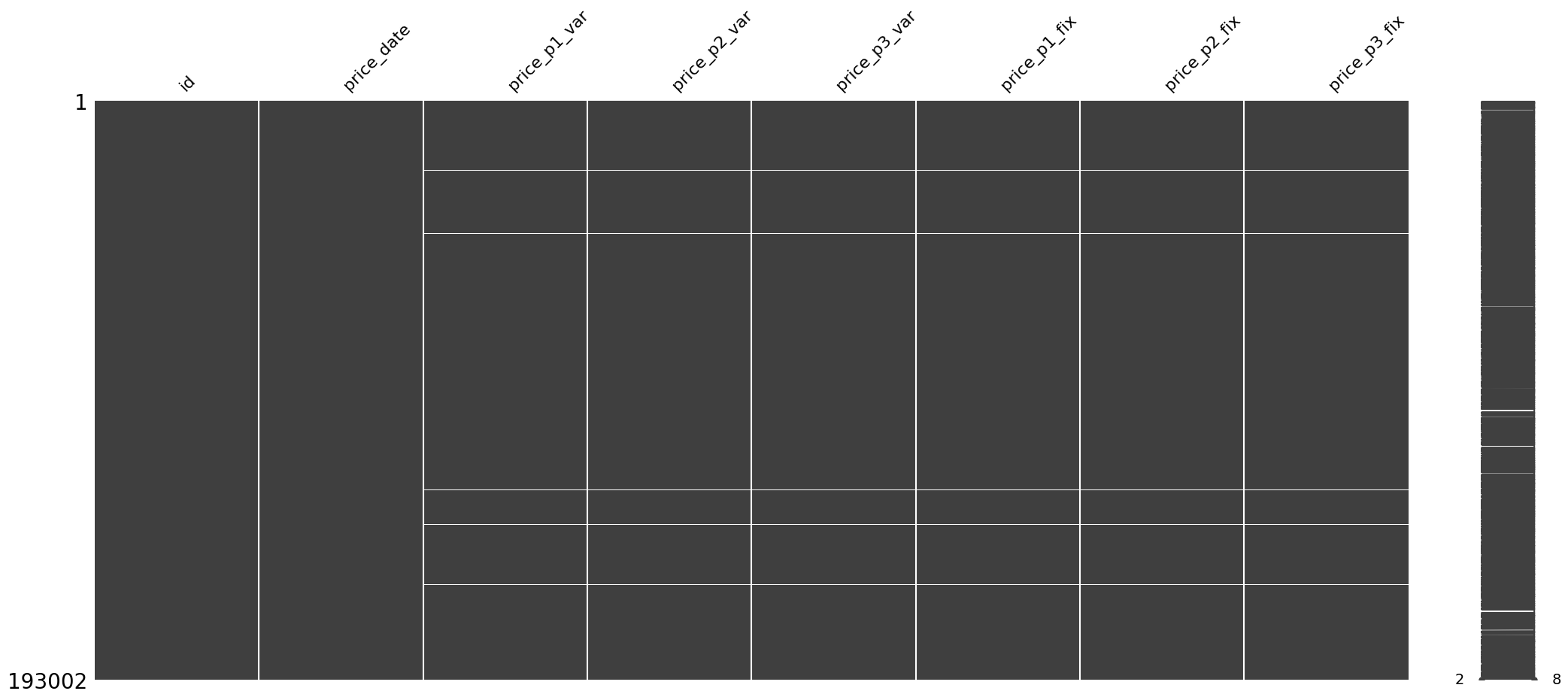
# Visualize the correlation between the numeric variables of the dataframe
msno.heatmap(pco_hist)
plt.show()
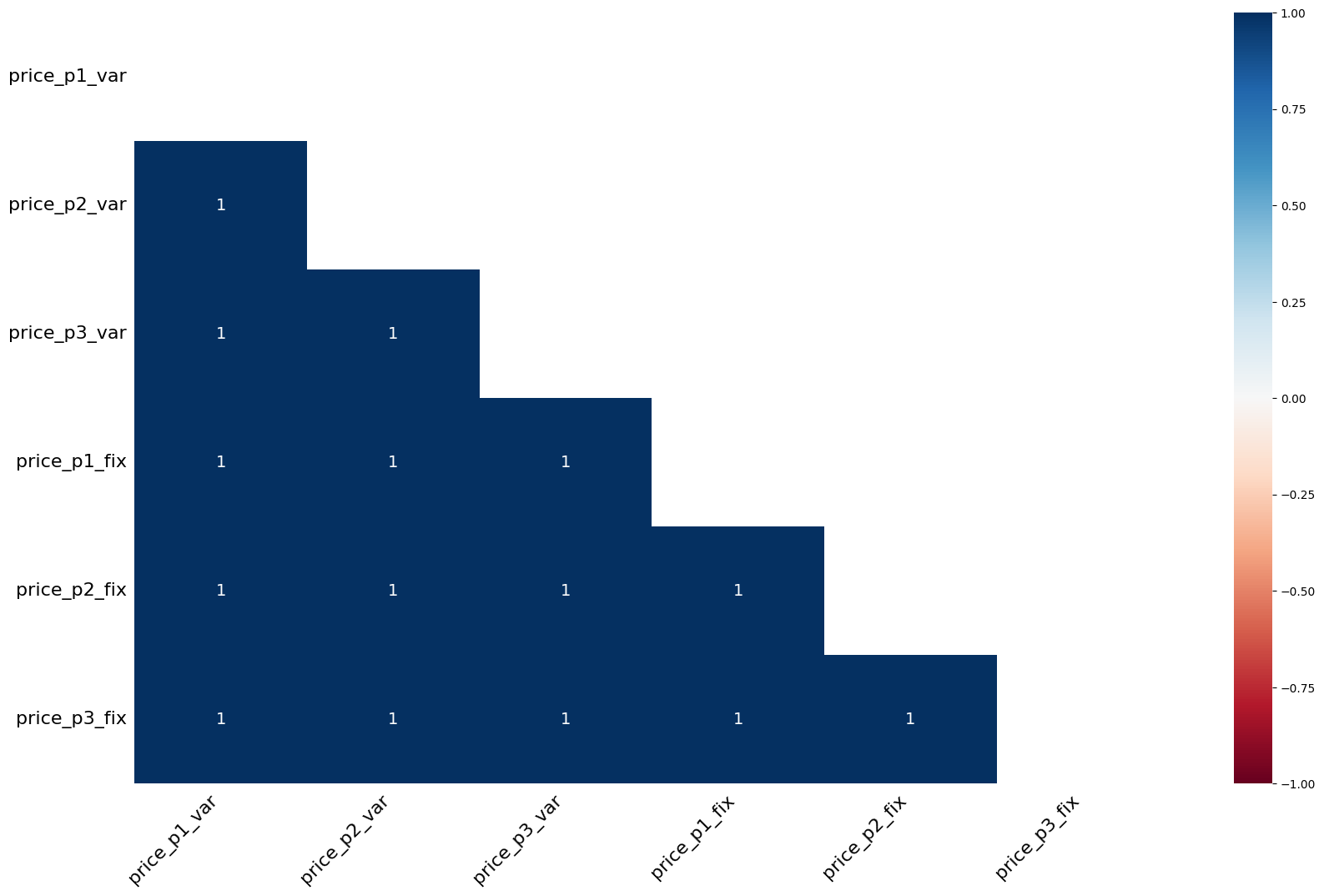
# Identify the index of the IDs containing missing values.
hist_NAN_index = pco_hist[pco_hist.isnull().any(axis=1)].index.values.tolist()
# Obtain a dataframe with the missing values
pco_hist_missing = pco_hist.iloc[hist_NAN_index,:]
# Glimpse at the NaN cases of the pco_hist dataset
pco_hist_missing.head(10)
id | price_date | price_p1_var | price_p2_var | price_p3_var | price_p1_fix | price_p2_fix | price_p3_fix | |
---|---|---|---|---|---|---|---|---|
75 | ef716222bbd97a8bdfcbb831e3575560 | 2015-04-01 | NaN | NaN | NaN | NaN | NaN | NaN |
221 | 0f5231100b2febab862f8dd8eaab3f43 | 2015-06-01 | NaN | NaN | NaN | NaN | NaN | NaN |
377 | 2f93639de582fadfbe3e86ce1c8d8f35 | 2015-06-01 | NaN | NaN | NaN | NaN | NaN | NaN |
413 | f83c1ab1ca1d1802bb1df4d72820243c | 2015-06-01 | NaN | NaN | NaN | NaN | NaN | NaN |
461 | 3076c6d4a060e12a049d1700d9b09cf3 | 2015-06-01 | NaN | NaN | NaN | NaN | NaN | NaN |
471 | 33bb3af90650ac2e9ecac6ff2c975a6b | 2015-04-01 | NaN | NaN | NaN | NaN | NaN | NaN |
472 | 33bb3af90650ac2e9ecac6ff2c975a6b | 2015-05-01 | NaN | NaN | NaN | NaN | NaN | NaN |
475 | 33bb3af90650ac2e9ecac6ff2c975a6b | 2015-08-01 | NaN | NaN | NaN | NaN | NaN | NaN |
476 | 33bb3af90650ac2e9ecac6ff2c975a6b | 2015-09-01 | NaN | NaN | NaN | NaN | NaN | NaN |
874 | 0e90101b08183cc9548e827e4b256f47 | 2015-12-01 | NaN | NaN | NaN | NaN | NaN | NaN |
# extract the unique dates of missing data
date_lst = pco_hist_missing['price_date'].unique()
id_lst = pco_hist_missing['id'].unique()
# Create a time dataframe with the unique dates
time_df = pd.DataFrame(data=date_lst, columns=['price_date'] )
# Glimpse the time dataframe
time_df.sort_values(by=['price_date'])
price_date | |
---|---|
9 | 2015-01-01 |
11 | 2015-02-01 |
8 | 2015-03-01 |
0 | 2015-04-01 |
2 | 2015-05-01 |
1 | 2015-06-01 |
10 | 2015-07-01 |
3 | 2015-08-01 |
4 | 2015-09-01 |
7 | 2015-10-01 |
6 | 2015-11-01 |
5 | 2015-12-01 |
Observations#
The columns containing prices display strong positive correlation in the missingness, suggesting a case of MNAR.
We can use trend and cyclicality when imputing time series data.
# Make a copy of pco_hist dataset
pco_hist_ff = pco_hist.copy(deep=True)
# Print prior to imputing missing values
print(pco_hist_ff.iloc[hist_NAN_index,3:9].head())
# Fill NaNs using forward fill
pco_hist_ff.fillna(method = 'ffill', inplace=True)
print(pco_hist_ff.iloc[hist_NAN_index,3:9].head())
price_p2_var price_p3_var price_p1_fix price_p2_fix price_p3_fix
75 NaN NaN NaN NaN NaN
221 NaN NaN NaN NaN NaN
377 NaN NaN NaN NaN NaN
413 NaN NaN NaN NaN NaN
461 NaN NaN NaN NaN NaN
price_p2_var price_p3_var price_p1_fix price_p2_fix price_p3_fix
75 0.000000 0.000000 44.266931 0.000000 0.000000
221 0.000000 0.000000 44.266931 0.000000 0.000000
377 0.087970 0.000000 44.266931 0.000000 0.000000
413 0.102239 0.070381 40.565969 24.339581 16.226389
461 0.000000 0.000000 44.266931 0.000000 0.000000
# Merge output dataset with historical forward fill dataset
pco_hist_ff_merged = pco_hist_ff.merge(right=pco_output,on=['id'])
pco_hist_ff_merged.head()
id | price_date | price_p1_var | price_p2_var | price_p3_var | price_p1_fix | price_p2_fix | price_p3_fix | churn | |
---|---|---|---|---|---|---|---|---|---|
0 | 038af19179925da21a25619c5a24b745 | 2015-01-01 | 0.151367 | 0.0 | 0.0 | 44.266931 | 0.0 | 0.0 | 0 |
1 | 038af19179925da21a25619c5a24b745 | 2015-02-01 | 0.151367 | 0.0 | 0.0 | 44.266931 | 0.0 | 0.0 | 0 |
2 | 038af19179925da21a25619c5a24b745 | 2015-03-01 | 0.151367 | 0.0 | 0.0 | 44.266931 | 0.0 | 0.0 | 0 |
3 | 038af19179925da21a25619c5a24b745 | 2015-04-01 | 0.149626 | 0.0 | 0.0 | 44.266931 | 0.0 | 0.0 | 0 |
4 | 038af19179925da21a25619c5a24b745 | 2015-05-01 | 0.149626 | 0.0 | 0.0 | 44.266931 | 0.0 | 0.0 | 0 |
The Main Dataset#
# Visualize the completeness of the dataframe
msno.bar(pco_main)
plt.show()
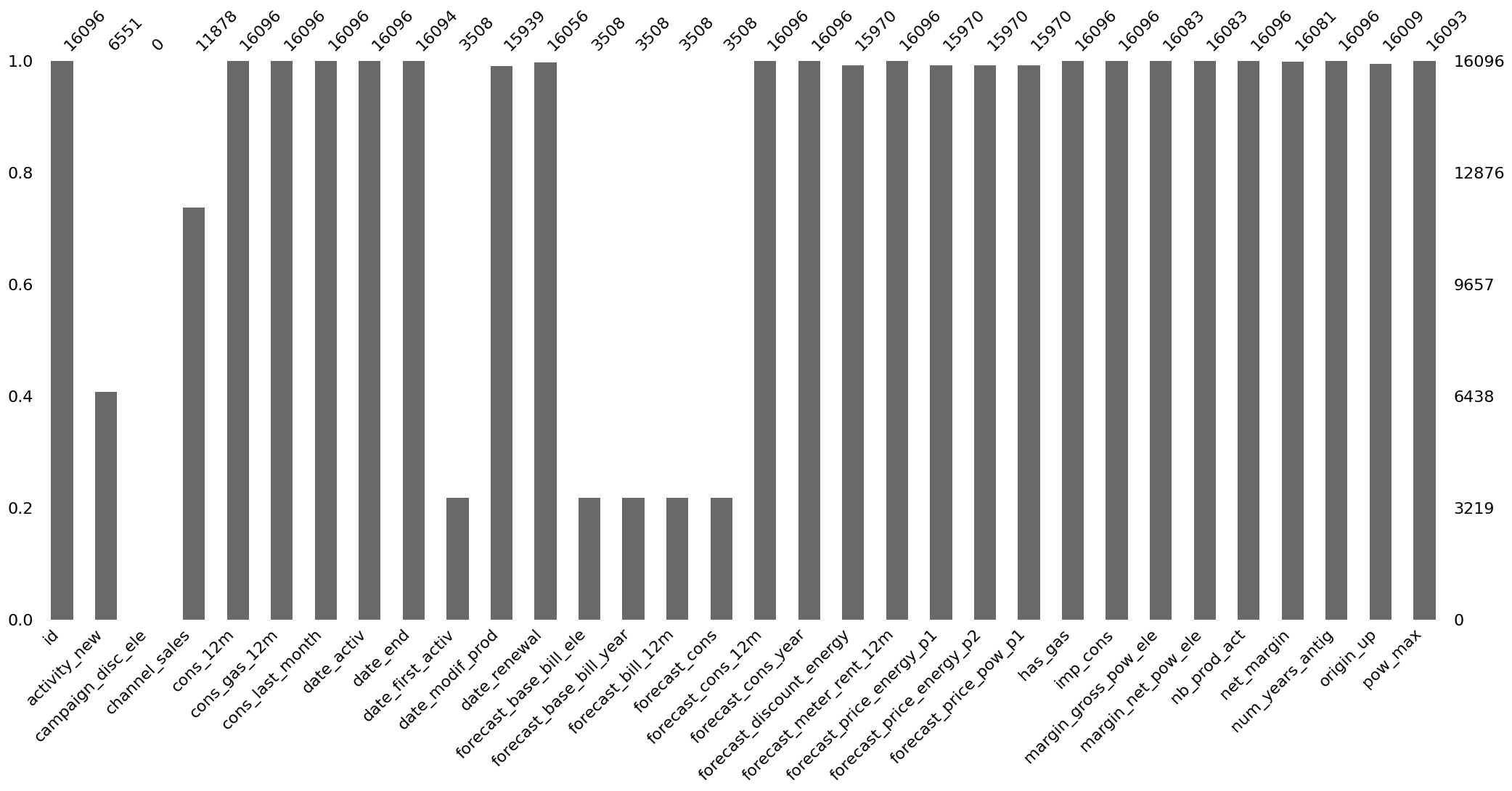
# Visualize the locations of the missing values of the dataset
msno.matrix(pco_main)
plt.show()
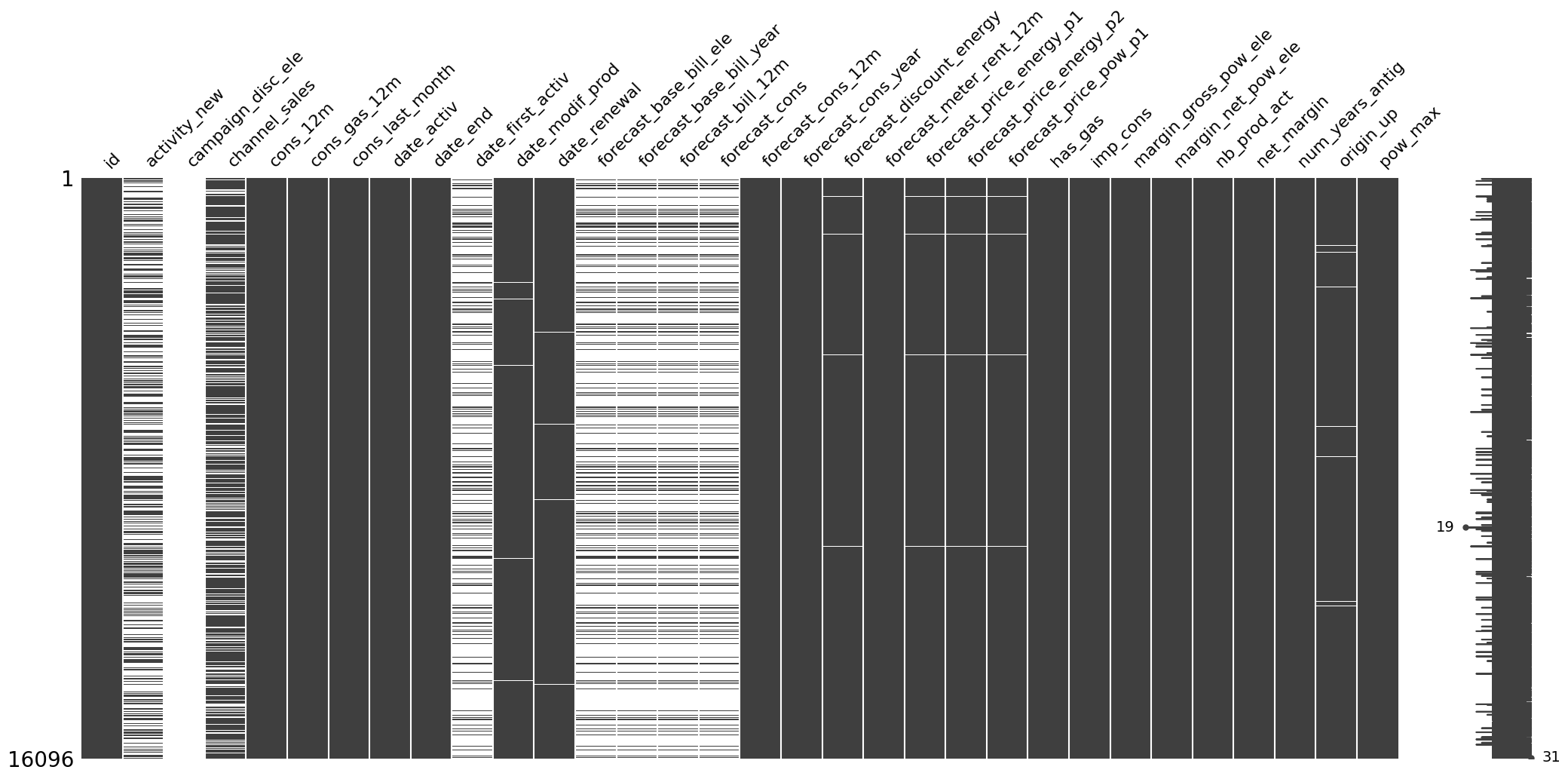
msno.heatmap(pco_main)
plt.show()
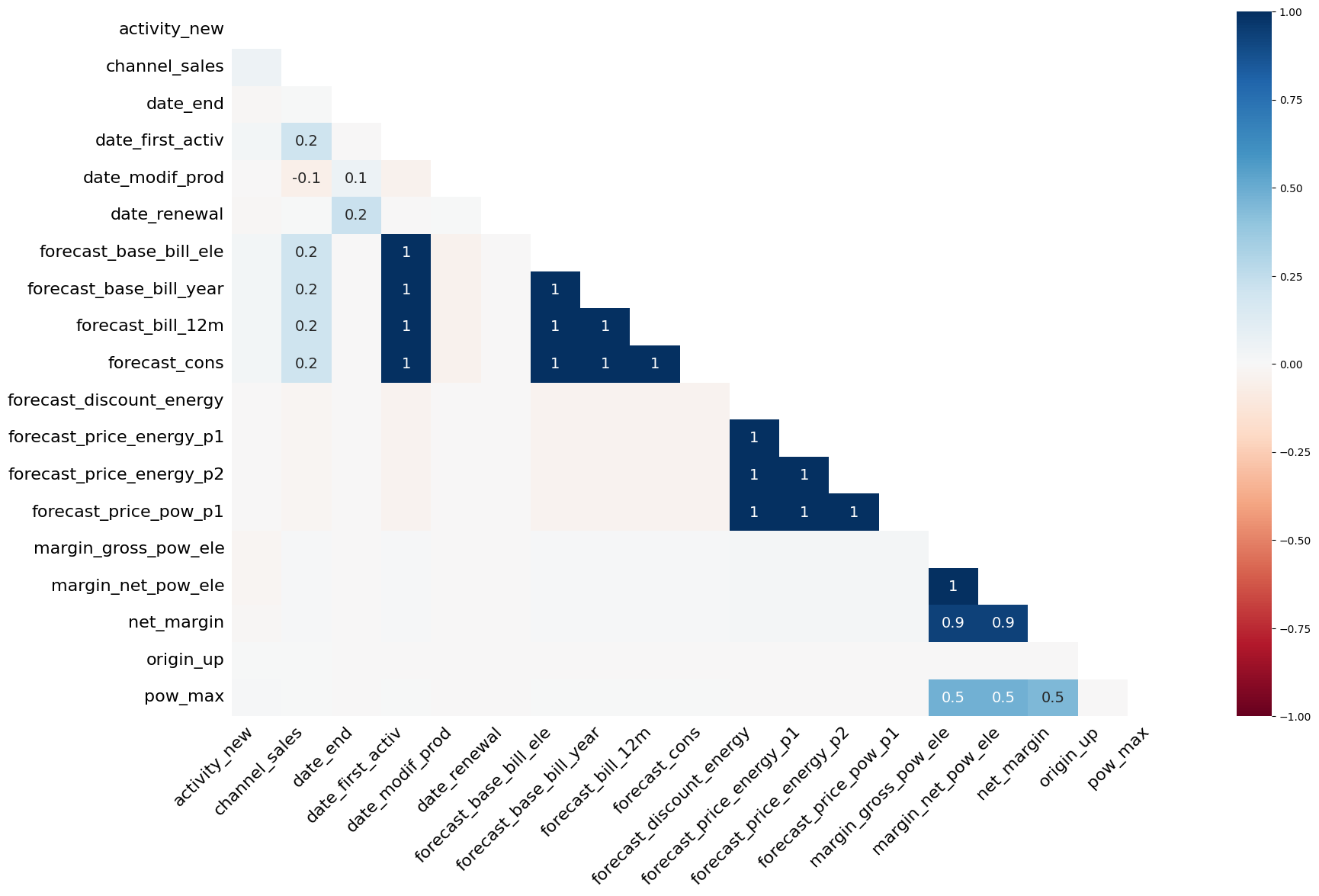
# Demonstrate why the date_activ column cannot replace completely date_first_activ
activity = ['date_activ','date_first_activ']
# Filter the columns of interest
pco_activity = pco_main[activity]
# Obtain only the complete cases
pco_activity_cc = pco_activity.dropna(subset=['date_first_activ'],how='any',inplace=False)
# Test whether two objects contain the same elements.
pco_activity_cc.date_activ.equals(pco_activity_cc.date_first_activ)
# Describe it
pco_activity_cc.describe()
date_activ | date_first_activ | |
---|---|---|
count | 3508 | 3508 |
mean | 2011-09-03 07:45:05.131128832 | 2011-06-19 20:20:23.261117440 |
min | 2003-09-23 00:00:00 | 2001-01-10 00:00:00 |
25% | 2010-10-26 00:00:00 | 2010-08-04 18:00:00 |
50% | 2012-01-03 00:00:00 | 2011-10-28 00:00:00 |
75% | 2012-08-08 00:00:00 | 2012-06-22 06:00:00 |
max | 2014-09-01 00:00:00 | 2014-09-01 00:00:00 |
# Drop the column activity_new and campaign_disc_elec
pco_main_drop = pco_main.drop(labels= ['activity_new','campaign_disc_ele'] , axis=1)
# Remove date_end date_modif_prod date_renewal origin_up pow_max margin_gross_pow_ele margin_net_pow_ele net_margin
brush = ['date_end','date_modif_prod','date_renewal','origin_up','pow_max','margin_gross_pow_ele',
'margin_net_pow_ele', 'net_margin','forecast_discount_energy','forecast_price_energy_p1',
'forecast_price_energy_p2','forecast_price_pow_p1']
pco_main_drop.dropna(subset=brush, how='any',inplace=True)
msno.matrix(pco_main_drop)
plt.show()
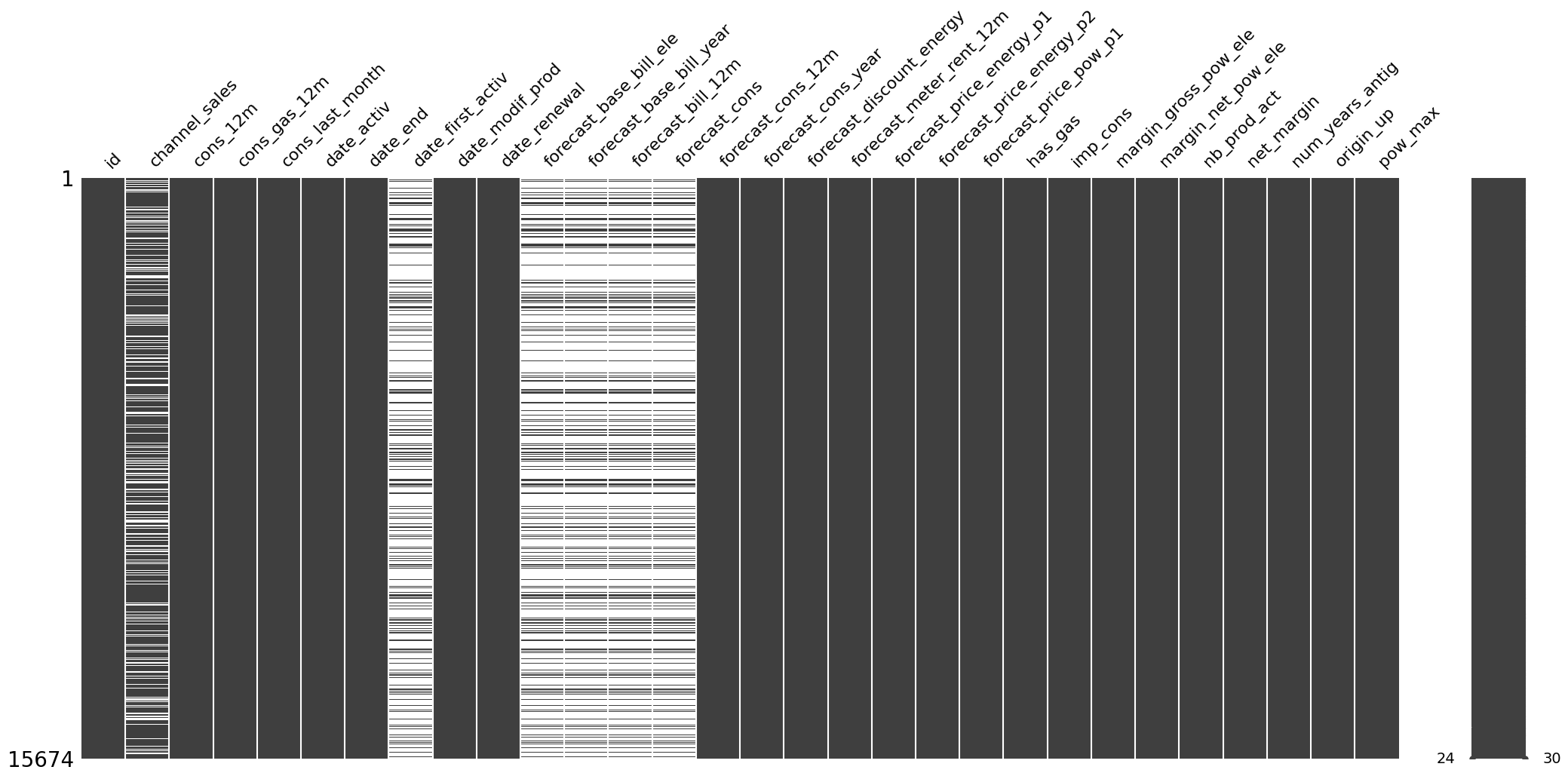
Observations#
activity_new
is MCAR with low correlation with other variables. Can drop this columncampaign_disc_elec
is MCAR. Can drop this column. Suggests that subscribers are not subscribing through campaign offers.date_first_activ
cannot replacedate_active
. MARnet_margin
has strong correlation betweenmargin_gross_pow_elec
andmargin)_net_pow_ele
. Suggests multi-colinearity.origin_up
andpow_max
is MCAR. Can drop.Forecast_base_bill_ele
,forecast_base_bill_year
,forecast_bill_12m
andforecast_cons variables
are highly correlated withdate_first_activ
. MNAR
# Choose the columns without missing values
incomplete_cols = ['channel_sales','date_first_activ','forecast_base_bill_ele','forecast_base_bill_year','forecast_bill_12m','forecast_cons']
complete_cols = [column_name for column_name in pco_main_drop.columns if column_name not in incomplete_cols]
pco_main_cc = pco_main_drop[complete_cols]
# Fix negative numeric variables
numeric = [column_name for column_name in pco_main_cc.columns
if pco_main_cc[column_name].dtype == 'float64'
or pco_main_cc[column_name].dtype == 'int64']
# Overwrite positive values on negative values
pco_main_cc[numeric] = pco_main_cc[numeric].apply(abs)
# Describe
pco_main_cc.describe()
cons_12m | cons_gas_12m | cons_last_month | date_activ | date_end | date_modif_prod | date_renewal | forecast_cons_12m | forecast_cons_year | forecast_discount_energy | forecast_meter_rent_12m | forecast_price_energy_p1 | forecast_price_energy_p2 | forecast_price_pow_p1 | imp_cons | margin_gross_pow_ele | margin_net_pow_ele | nb_prod_act | net_margin | num_years_antig | pow_max | |
---|---|---|---|---|---|---|---|---|---|---|---|---|---|---|---|---|---|---|---|---|---|
count | 1.567400e+04 | 1.567400e+04 | 1.567400e+04 | 15674 | 15674 | 15674 | 15674 | 15674.000000 | 15674.000000 | 15674.000000 | 15674.000000 | 15674.000000 | 15674.000000 | 15674.000000 | 15674.000000 | 15674.000000 | 15674.000000 | 15674.000000 | 15674.000000 | 15674.000000 | 15674.000000 |
mean | 1.916143e+05 | 3.132400e+04 | 1.941588e+04 | 2011-01-08 05:05:17.417379328 | 2016-07-28 01:39:57.396962816 | 2012-12-09 11:44:28.419037952 | 2015-07-21 02:51:14.952150016 | 2359.676441 | 1911.698354 | 0.976139 | 70.210965 | 0.135925 | 0.052858 | 43.522191 | 196.641669 | 23.556272 | 24.125235 | 1.348092 | 221.259158 | 5.052188 | 20.438270 |
min | 0.000000e+00 | 0.000000e+00 | 0.000000e+00 | 2000-07-25 00:00:00 | 2013-05-06 00:00:00 | 2000-07-25 00:00:00 | 2013-06-26 00:00:00 | 0.000000 | 0.000000 | 0.000000 | 0.000000 | 0.000000 | 0.000000 | 0.000000 | 0.000000 | 0.000000 | 0.000000 | 1.000000 | 0.000000 | 1.000000 | 1.000000 |
25% | 5.893250e+03 | 0.000000e+00 | 0.000000e+00 | 2010-01-11 00:00:00 | 2016-04-27 00:00:00 | 2010-08-04 00:00:00 | 2015-04-17 00:00:00 | 514.045000 | 0.000000 | 0.000000 | 16.230000 | 0.115237 | 0.000000 | 40.606701 | 0.000000 | 12.360000 | 12.360000 | 1.000000 | 52.802500 | 4.000000 | 12.500000 |
50% | 1.522000e+04 | 0.000000e+00 | 9.090000e+02 | 2011-02-21 00:00:00 | 2016-08-01 00:00:00 | 2013-04-22 00:00:00 | 2015-07-27 00:00:00 | 1178.970000 | 382.000000 | 0.000000 | 19.430000 | 0.142881 | 0.086163 | 44.311378 | 44.870000 | 21.090000 | 21.090000 | 1.000000 | 120.545000 | 5.000000 | 13.856000 |
75% | 4.953825e+04 | 0.000000e+00 | 4.131500e+03 | 2012-04-17 00:00:00 | 2016-11-01 00:00:00 | 2015-05-24 00:00:00 | 2015-10-30 00:00:00 | 2677.220000 | 1994.750000 | 0.000000 | 131.500000 | 0.146348 | 0.098837 | 44.311378 | 217.962500 | 29.640000 | 29.760000 | 1.000000 | 275.797500 | 6.000000 | 19.800000 |
max | 1.609711e+07 | 4.154590e+06 | 4.538720e+06 | 2014-09-01 00:00:00 | 2017-06-13 00:00:00 | 2016-01-29 00:00:00 | 2016-01-28 00:00:00 | 103801.930000 | 175375.000000 | 50.000000 | 2411.690000 | 0.273963 | 0.195975 | 59.444710 | 15042.790000 | 525.540000 | 615.660000 | 32.000000 | 24570.650000 | 16.000000 | 500.000000 |
std | 6.724688e+05 | 1.716291e+05 | 8.226881e+04 | NaN | NaN | NaN | NaN | 3979.605687 | 5224.813531 | 5.124103 | 78.560454 | 0.026282 | 0.048638 | 5.221651 | 490.956048 | 22.456277 | 25.599218 | 1.475092 | 362.053657 | 1.670284 | 21.164053 |
# Convert the has_gas column to Yes/No
pco_main_cc['has_gas'] = pco_main_cc['has_gas'].replace({'t':'Yes','f':'No'})
# Merge the main dataset with the output dataset
pco_main_cc_merged = pco_main_cc.merge(right=pco_output,on=['id'])
# Convert the churn column to Churned/Stayed
pco_main_cc_merged['churn'] = pco_main_cc_merged['churn'].replace({1:'Churned',0:'Stayed'})
pco_main_cc_merged.head()
id | cons_12m | cons_gas_12m | cons_last_month | date_activ | date_end | date_modif_prod | date_renewal | forecast_cons_12m | forecast_cons_year | forecast_discount_energy | forecast_meter_rent_12m | forecast_price_energy_p1 | forecast_price_energy_p2 | forecast_price_pow_p1 | has_gas | imp_cons | margin_gross_pow_ele | margin_net_pow_ele | nb_prod_act | net_margin | num_years_antig | origin_up | pow_max | churn | |
---|---|---|---|---|---|---|---|---|---|---|---|---|---|---|---|---|---|---|---|---|---|---|---|---|---|
0 | 48ada52261e7cf58715202705a0451c9 | 309275 | 0 | 10025 | 2012-11-07 | 2016-11-06 | 2012-11-07 | 2015-11-09 | 26520.30 | 10025 | 0.0 | 359.29 | 0.095919 | 0.088347 | 58.995952 | No | 831.80 | 41.76 | 41.76 | 1 | 1732.36 | 3 | ldkssxwpmemidmecebumciepifcamkci | 180.000 | Stayed |
1 | d29c2c54acc38ff3c0614d0a653813dd | 4660 | 0 | 0 | 2009-08-21 | 2016-08-30 | 2009-08-21 | 2015-08-31 | 189.95 | 0 | 0.0 | 16.27 | 0.145711 | 0.000000 | 44.311378 | No | 0.00 | 16.38 | 16.38 | 1 | 18.89 | 6 | kamkkxfxxuwbdslkwifmmcsiusiuosws | 13.800 | Stayed |
2 | 764c75f661154dac3a6c254cd082ea7d | 544 | 0 | 0 | 2010-04-16 | 2016-04-16 | 2010-04-16 | 2015-04-17 | 47.96 | 0 | 0.0 | 38.72 | 0.165794 | 0.087899 | 44.311378 | No | 0.00 | 28.60 | 28.60 | 1 | 6.60 | 6 | kamkkxfxxuwbdslkwifmmcsiusiuosws | 13.856 | Stayed |
3 | bba03439a292a1e166f80264c16191cb | 1584 | 0 | 0 | 2010-03-30 | 2016-03-30 | 2010-03-30 | 2015-03-31 | 240.04 | 0 | 0.0 | 19.83 | 0.146694 | 0.000000 | 44.311378 | No | 0.00 | 30.22 | 30.22 | 1 | 25.46 | 6 | kamkkxfxxuwbdslkwifmmcsiusiuosws | 13.200 | Stayed |
4 | 568bb38a1afd7c0fc49c77b3789b59a3 | 121335 | 0 | 12400 | 2010-04-08 | 2016-04-08 | 2010-04-08 | 2015-04-12 | 10865.02 | 12400 | 0.0 | 170.74 | 0.110083 | 0.093746 | 40.606701 | No | 1052.37 | 3.18 | 3.18 | 1 | 823.18 | 6 | lxidpiddsbxsbosboudacockeimpuepw | 75.000 | Stayed |
# Obtain all the variables except for id
variables = [column_name for column_name in pco_main_cc_merged.columns if column_name != 'id']
# Obtain all the categorical variables except for id
categorical = [column_name for column_name in variables if pco_main_cc_merged[column_name].dtype == 'object']
# Obtain all the Date Variables
dates = [column_name for column_name in variables if pco_main_cc_merged[column_name].dtype == 'datetime64[ns]']
# Obtain all the numeric columns
numeric = [column_name for column_name in variables
if column_name not in categorical
and column_name != 'id'
and column_name != 'churn'
and column_name not in dates]
Data Visualization#
The Output Dataset#
# Calculate the zcores of tenure
tenure_zcores = zscore(a=pco_main_cc_merged['num_years_antig'])
# Convert to absolute values
abs_tenure_zscores = np.abs(tenure_zcores)
# Extract Columns of interest
churn_tenure = pco_main_cc_merged[['churn','num_years_antig']]
# Add z-score column
churn_tenure['z_score'] = list(abs_tenure_zscores)
# Remove outliers
churned_tenure_filtered = churn_tenure[churn_tenure['z_score'] < 3]
# Visualize tenure by retained customer and churner
vio = sns.violinplot( y=churned_tenure_filtered["churn"], x=churned_tenure_filtered["num_years_antig"] )
# Settings
vio.set(xlabel='Years', ylabel='')
vio.set_title("Customer Attrition by Tenure")
plt.show()
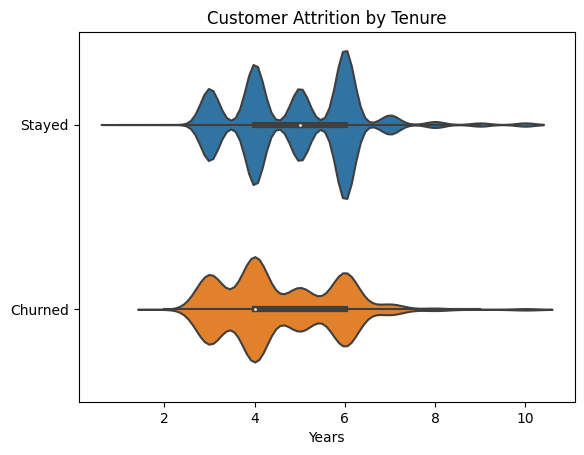
Facts#
The median age of churners is 4 years
Customers are more likely to churn during the 4th year than the 7th year
The median age of retained customers is 5 years
The Main Dataset#
# Most popular electricity campaign in the dataset
ele_nm = pco_main_cc_merged.loc[(pco_main_cc_merged['churn']>='Stayed') & (pco_main_cc_merged['net_margin']>0),['id', 'origin_up','net_margin']]
ele_nm.groupby('origin_up')['net_margin'].count().sort_values(ascending=False)
origin_up
lxidpiddsbxsbosboudacockeimpuepw 6584
kamkkxfxxuwbdslkwifmmcsiusiuosws 4188
ldkssxwpmemidmecebumciepifcamkci 3201
usapbepcfoloekilkwsdiboslwaxobdp 2
ewxeelcelemmiwuafmddpobolfuxioce 1
Name: net_margin, dtype: int64
# Highest netting electricity subscription campaign
print(ele_nm.groupby('origin_up')['net_margin'].agg('sum').sort_values(ascending=False))
origin_up
lxidpiddsbxsbosboudacockeimpuepw 1541159.95
ldkssxwpmemidmecebumciepifcamkci 814230.02
kamkkxfxxuwbdslkwifmmcsiusiuosws 717939.95
usapbepcfoloekilkwsdiboslwaxobdp 250.40
ewxeelcelemmiwuafmddpobolfuxioce 46.22
Name: net_margin, dtype: float64
Facts#
The most popular electricity campaign is
lxidpiddsbxsbosboudacockeimpuepw
which has brought 6,584 current customers. With a net margin of $1,541,159.95 in 2015.
# Select current customers with positive net margins
top_customers = pco_main_cc_merged.loc[(pco_main_cc_merged['churn']>='Stayed') & (pco_main_cc_merged['net_margin']>0),['id','num_years_antig','net_margin']]
# Top 10 customers by net margin
top_customers.sort_values(by=['net_margin'],ascending=False).head(10)
id | num_years_antig | net_margin | |
---|---|---|---|
11502 | d00e8a9951b5551d8f02e45f9ed2b0dd | 3 | 10203.50 |
6930 | 78bd1c5c0c67f2be6de89b19df5f8861 | 3 | 5625.14 |
13259 | 818b8bca0a9d7668252d46b978169325 | 4 | 4346.37 |
8378 | a3a739686fbd5ba8b4a21ec835507b6d | 4 | 4305.79 |
324 | 89b3406c3ba717f1b788ceeb5af9e8b9 | 3 | 4161.74 |
10100 | 93435ecb05910c7b87e0ae9dbedb2882 | 4 | 4148.99 |
12028 | 4519e6a8928a015819466fc9de0fa49e | 3 | 4040.60 |
6405 | 933527d7a2f669af49075a2380c10ded | 4 | 3744.72 |
6850 | 43580ef6cc40fcfd0a9b76eee17a267a | 4 | 3716.78 |
13553 | ee98a86efa759681cc59c7d4e0d0312f | 4 | 3407.65 |
These are the most profitable customers for PowerCo in terms of net margin. Notet that most of them are within the likely tenure of attrition.
#!jupyter-nbconvert --to PDFviaHTML BCG.ipynb